Optimizing E&P using strategic criteria and new geophysical technologies
The selection and combination of seismic attributes (defined as any measurable property of seismic data) used during the interpretation process is vital to identifying geometric patterns. This results in large amounts of data, but when properly handled, it creates a sharper, more useful image of the subsurface. This decreases uncertainty and provides more precise approximation to static models of the reservoir and improves understanding of the tectonic and stratigraphic mechanisms. The application of new techniques, as multiple realistic co-visualizations (MRC), big data analysis, and machine learning strategies, are improving the quality of results when compared to those obtained with traditional workflows.
Multi-attribute realistic co-visualizations. The technological evolution of interpretation systems, and the availability of enhanced seismic processing routines, has enabled geophysicists to incorporate algorithms linked to geometric and physical properties of seismic data. This increases the possibility of calculating attributes, and the possibility of generating helpful visual expressions.
The multiple realistic co-visualization technique is a valuable and effective tool to make key decisions in the interpretation stage. MRC is defined as the combination and assembly of different attributes to enrich subsurface interpretable images with greater confidence. Using MRC reinforces seismic resolution and, therefore aids in identifying geological expressions of vertical and horizontal features of smaller size and resolution.
The MRC techniques are a new, realistic approximation to seismic, that helps engineers to visualize and derive feasible, prospective alternatives in the analysis and interpretation stages. These techniques also allow interpreters to evaluate the impact of the multiple co-visualizations of attributes in their structural and stratigraphic situations, and obtain better definition of fault planes, seismic subvertical features and associated geological structures like dikes and domes.
Since most seismic interpreters use traditional, generally-designed workflows, they need focused criteria and clear objectives to derive a more efficient interpretation sequence. The routines applied to the complex structural analysis, supported by automatic structural events recognition tools, are included in the different interpretation systems. But by broadening the analysis criteria, it is possible to identify improvements in the interpretation tools, such as attribute calculation, special processing, seismic inversion, mapping, contouring and visualizations.1
One of the most striking attributes to achieve a clear and convincing identification of seismic architectural elements, is by using a high-frequency version of the amplitude volume technique (AVThf).2 Through this interpretive tool, combined with MRC techniques, it is possible to define, with confidence, stratigraphic events, faults, relative sequence order and the relationship between architectural elements of deposition/reservoirs and other components of the interpretive subsurface model.
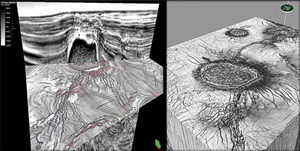
The basic amplitude volume technique (AVT) attribute enables a wide range of possibilities of representation, interpretation, and analysis. It forms the basis of the calculation flow to obtain an attribute variant with higher-frequency content meta-attributes along with visualization as variants of the AVT, Fig. 1. The enhanced interpretation highlights the subtle stratigraphic and structural features that tend to be difficult to identify in seismic amplitudes. They were subsequently identified as a diverse grade of complex fault systems, subvertical seismic events, reflectors discontinuities with several geometric patterns and different types of unconformities.
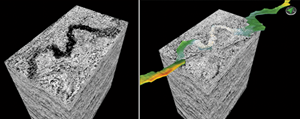
Although, these substantial improvements aid interpretation, the calculation of meta-attributes (an attribute derived from another), the application of oriented structural filters and the use of automatic fault recognition tools make a superlative contribution for the best positioning of geological structures, both vertically and horizontally. As such, it is advisable to generate several multi-attribute co-visualizations of all available information to find the one that best suits the desirable interpretation, Fig. 2.3,4
The improved visualizations increase the knowledge of the geological history of a frontier region to help delineate a new prospect and in the reinterpretation of a known area to determine its production limits.
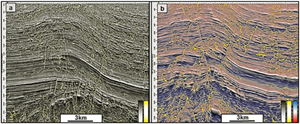
Figure 3a shows how the MRC process is used to visualize and interpret fault systems. In this case, a faulted and thrusted belt was identified through the co-visualization of AVThf attribute, combined with an automatic fault recognition process. Back-thrust and different-order associated faults can be interpreted. Figure 3b shows this in a similar structural style, but with the addition of the instantaneous phase complex trace attribute with AVThf as input. The clear recognition of more architectural elements considerably improves the final seismic model of the studied area.
Big data and machine learning challenges. The industry has traditionally collected large volumes of different types of information, including semi-structured and unstructured types. But by adopting new IT solutions designed to address specific challenges,5 it’s now possible to manage and analyze these growing volumes of data.
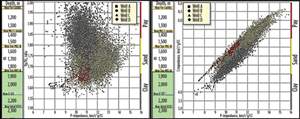
Using machine learning technologies, it is possible to apply artificial intelligence (AI) algorithms that provide to different workflow systems the capability to automatically learn and improve from experience. Machine learning focuses on the development of computer programs that can access data and learn from its application. Machine learning refers to the changes in the systems that perform tasks associated with AI. This routine involves recognition, diagnosis, planning, control and prediction of changes to enhance established systems or support the start-up of new ones, Fig. 4.
The most important part of the machine learning process is to understand the nature of the data and how it relates to solving a specific task. The process of learning begins with observations to determine patterns to make better decisions, based on the examples provided. The primary aim is to enable computers to learn automatically, with minimal human assistance, by adjusting actions according to targets. In this way, the modeling and simulation processes can be accelerated, and a large number of options evaluated, simultaneously.
In many cases, important relationships and correlations are hidden among large amounts of data. Machine learning methods can often be used to extract these connecting links. However, it is important to understand that each algorithm is uniquely tailored for data types to solve a specific problem. Visualizing the context of a problem, the algorithms and methods in machine learning are only one part of a greater process to solve a problem. As such, it’s advantageous to keep the big picture in mind during the exploration process.
Digital transformation. Over the past two decades, the oil and gas industry has seen significant advancements in interpretation capabilities, driven by increased computing power and new visualization technology. Advanced picking and tracking algorithms, used in seismic interpretation software, are now part of a seismic interpreter’s toolkit. These programs can identify horizons and faults and be used for integration of pre-stack and post-stack seismic data. They also provide detailed mapping capabilities, integration of well data, development of static geologic models and generation of SAS.
Data processing challenge. A significant issue in today’s interpretation environment is the enormous amount of data that is used and generated in, and for, our workstations. Seismic gathers, regional 3D surveys with numerous processing versions, large populations of wells and associated data, hundreds of SAS, routinely produce terabytes of data. The ability for the interpreter to make meaningful interpretations from this massive amount of data can be difficult and sometimes inefficient. Is the next step in the advancement of interpretation the ability to process large quantities of seismic data more effectively and derive more meaningful information from the data? We say yes!
To solve the big data challenge, we propose using AI, data analytics and new digital technologies, but for different purposes. We have accomplished seismic facies classification using attributes, through unsupervised or supervised learning and clustering, fault/horizon interpretation and geological features, as in well-log correlation.
Over the last several years, interpreters have been working on more powerful workstations and applying numerous new algorithms to identify seismic attributes (SAS). There have also been efforts to manage the large amount of calculations into volumes that can be evaluated for their geologic meaning and improved seismic interpretation. Commonly used categories include instantaneous, geometric, amplitude accentuating, amplitude-variation with offset, spectral decomposition and inversion.6
This new approach to identify SAS incorporates principal component analysis (PCA). PCA is a linear quantitative technique proven to significantly enhance the understanding of which SAS, or combination of attributes have interpretive significance. PCA reduces a large data set to indicate variations in the information, which often relate to geologic features of interest. PCA, as a tool used in an interpretation workflow, can help to determine meaningful seismic attributes.
The interpreter should think about which attributes should be used to differentiate a given seismic facies from the general background, or to differentiate multiple or complex facies among them. Experienced interpreters are adept in identifying attributes related to reflector continuity, wavelet morphology, spectrum, and energy. Using these criteria, interpreters must choose the optimal attribute, also as a function of the seismic data quality and relate to seismic facies, that the interpreter wants to isolate.
In turn, these attributes from PCA are input to self-organizing-map (SOM) training. The SOM is a form of unsupervised neural networks, which has proven to work together with these SAS to produce meaningful and easily interpretable results. SOM analysis reveals the natural clustering and patterns in data. It has been beneficial in defining stratigraphy, seismic facies, direct hydrocarbon indicator features when working in conventional areas, and other aspects in shale plays, such as fault/fracture trends and sweet spots.
These techniques revitalize the seismic events characterization and will make the interpretative effort more efficient, generating a clearer resolution of the models required for E&P modeling. The generation of reliable images on a sound physical basis, taking into account all available data types, will provide a new vision to perform multidisciplinary analysis to encourage creativity and teamwork, which will produce more effective results.
These new technologies enable users to integrate the results and conclusions of multidisciplinary studies, to maximize their use and optimize the knowledge of reservoir resources. The technique also makes it possible to quantify and analyze uncertainty, calculate original volumes of HC in situ, and perform more realistic and trustworthy fluid simulation. Additionally, it is also possible to strengthen field development plans and to statistically evaluate the static connectivity in the 3D volume of a reservoir, and identify in advance the areas with bypassed oil for infill drilling.
CONCLUSION
The multiple, realistic co-visualization technique is a valuable and effective tool to make key decisions in the interpretation stage. Using MRC has made it possible to reinforce seismic resolution to identify geometries associated with geological events in areas of prospective interest.
Through the evaluation of results, using different interpretation tools and special seismic processes with specific targets, it is possible to recognize patterns of interest in reservoirs that can be detected and visualized in terms of their geometry, orientation, extension, thickness and position in the stratigraphic column.
A comprehensive criterion is to use big data analysis and machine learning implementation by integrating detailed correlation between wells, velocity modeling, spectral decomposition and seismic attributes to detect (and/or visualize) such reservoirs. The analysis also establishes reservoir geometry, orientation, extension, stratigraphic position and other static and dynamic characterization parameters.
It is advantageous to look for new perspectives in data analysis when attempting to map and fully understand the subsurface geology in a frontier area. The first interpretation of the data can be deceptive and influenced by previous modeling exercises. In most cases, it is a best-practice to analyze the complete data set to increase the probability of success and encourage development of different applications by multidisciplinary teams.
In this sense, it has been shown that the application of tools and methodologies that aim to identify mineralized reservoirs and/or the sequences that contain them is helpful in development areas. The election of the appropriate interpretation tool, as described above, enables geophysicists to understand the sedimentary systems and their associated structural schemes. This is one of the most important elections of seismic interpreters, especially in highly-complex geological environments. Early and effective detection of potential reservoirs optimizes a project’s profitability.
ACKNOWLEDGEMENTS
The authors would like to thank Pan American Energy for permission to publish the examples of this job and to Ignacio Rovira for his technical contribution.
REFERENCES
- Chopra S., K.J. Marfurt, “Getting more from frequency-enhanced data,” Interpreter´s Corner, Explorer, AAPG, March 2011.
- Vernengo, L., E. Trinchero, M. García Torrejón and I. Rovira, “Amplitude volume technique attributes and multidimensional seismic interpretation,” The Leading Edge, SEG, pp. 308-313, September 2017.
- Vernengo, L., E. Trinchero, “Virtuous circle of seismic interpretation attributes,” Geohorizons, SPG (India), pp. 24-28, November 2017.
- Vernengo, L., E. Trinchero, M. García Torrejón and I. Rovira “Attributes at your fingertips, explorer,” Interpreter´s Corner, AAPG, pp. 22-26, November 2017.
- Holdaway, K., “Harness oil and gas big data with analytics,” John Wiley & Sons, New Jersey, USA, pp., 2014.
- Infante-Paez, L. and K. Marfurt, “Using machine learning as an aid to seismic geomorphology, which attributes are the best input?” Interpretation, SEG and AAPG, pp. 1-58, 2019.
- Digital transformation/Late-life optimization: Harnessing data-driven strategies for late-life optimization (March 2024)
- The reserves replacement dilemma: Can intelligent digital technologies fill the supply gap? (March 2024)
- Digital tool kit enhances real-time decision-making to improve drilling efficiency and performance (February 2024)
- Digital transformation: Digital twins help to make the invisible, visible in Indonesia’s energy industry (January 2024)
- Digital transformation: A breakthrough year for digitalization in the offshore sector (January 2024)
- Quantum computing and subsurface prediction (January 2024)
- Applying ultra-deep LWD resistivity technology successfully in a SAGD operation (May 2019)
- Adoption of wireless intelligent completions advances (May 2019)
- Majors double down as takeaway crunch eases (April 2019)
- What’s new in well logging and formation evaluation (April 2019)
- Qualification of a 20,000-psi subsea BOP: A collaborative approach (February 2019)
- ConocoPhillips’ Greg Leveille sees rapid trajectory of technical advancement continuing (February 2019)