Balancing AI advances with robust cybersecurity solutions
Advances in the oil and gas industry over the past decade have included a rapid digitization of operating technologies and the introduction of artificial intelligence (AI) to optimize newly automated systems. At the same time, the industry has experienced dramatic changes in the way it works, most notably during the past year, as Covid-19 ushered in a new era of remote work. Also, lower commodity prices and increased pressure to lessen the industry’s environmental impact while increasing operating efficiencies to compensate for market pressures also factored into the transition requirement.
These forces combined to push the industry to quickly implement new digital solutions, to enable employees to work from home, reduce our carbon footprint and streamline operations to improve the bottom line. But with this wealth of new opportunities, digitization also brought a need for enhanced cybersecurity to monitor and protect the smart infrastructure that is now present throughout every facet of oil and gas development, from the reservoir to the refinery, Fig. 1.
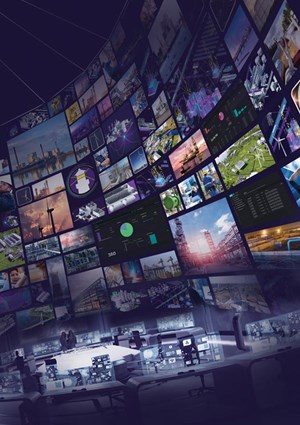
How exactly is AI affecting oil and gas companies’ digital transformation and cybersecurity strategies in their daily operations? A recent MIT Technology Review Insights report, created in association with Siemens Energy, aimed to find answers to this critical question. Interviews with more than a dozen information technology (IT) and cybersecurity executives at oil and gas companies around the world uncovered several key findings.
First, many companies confirm that they need to adopt digital technologies to help them pivot to new, streamlined ways of working. But as they add digital technologies to expand data collection and analysis, connect equipment to the internet of things, and improve their forecasting to boost profits, they are making their IT and operational technology (OT) more vulnerable to cyberattacks. As a result, companies need to continually improve their cyber resilience to detect and prevent attacks, and to withstand and recover from those that do occur. To respond to the threat, companies have found that cybersecurity tools, powered by AI and machine learning, must be at the forefront of every aspect of their digital transformation strategies.
Collaborate to innovate. On the road to the digital transition, the MIT report confirmed that oil and gas companies are living in a combined era of hyperconnectivity and mega attacks that can have devastating consequences for the productivity and profitability of their operations. And with the gap between cyberattackers and defenders continuing to expand with the increasing sophistication and frequency of attacks, how can the industry hope to catch up? For some larger energy companies, the answer lies in building and deploying their own OT cybersecurity solutions. But many small-to-mid-sized companies realize that their solutions rely on developing unique partnerships that tap into the promise of AI by building built-for-purpose solutions that make AI practical and easier to implement. And, because many companies don’t have the budget or sufficient in-house resources to build the required AI on their own, they are opening the innovation cycle by collaborating with partners who can provide the expertise and support required, as the threat environment evolves.
Specifically, a three-legged stool of companies comprising AI startups, original equipment manufacturers (OEMs), and operators can more effectively tap into and enhance AI technologies to build solutions that address obsolescence, intermittent connectivity, workflows, and a focus on safety and reliability.
Partnerships between original equipment manufacturers and tech vendors are often where the real innovation for industry-specific cybersecurity happens. As an example, Siemens Energy recently collaborated with AI startup SparkCognition to protect fleets of assets, including power generators and pipelines. They developed DeepArmor Industrial, a perpetually vigilant cybersecurity product that uses AI to flag cyberthreats against energy assets before an attack occurs, such as using machine learning to figure out why a program might turn into malware and then defend against it.
The AI-driven production platform is different from existing endpoint solutions, in that it provides a layer of defense that is independent of threat intelligence, while eliminating the need for threat signature updates or specialized analysts to secure individual OT systems. The platform uses behavioral analysis to continuously monitor and detect new threats, to provide a first line of defense and immediate protection against both previously identified and zero-day attacks on endpoint devices. As a result, the platform can prevent or mitigate attacks from new sources that are still unknown to the industry or security professionals, while lowering the security spend for asset owners.
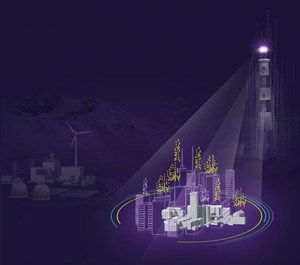
Proving its potential with a tailored defense approach. DeepArmor Industrial’s intelligent defense and detection system, customized design, and site-specific deployment can help provide reliable and robust cybersecurity for brownfield and digitally native assets alike. Consider compressor stations, which might have been cutting-edge when deployed decades ago but have been undermaintained since their commissioning. Some of these older stations, which are in remote locations along a pipeline, rely on a combination of analog equipment and digital retrofits, Fig. 2. Depending on the equipment, the stations might only connect to networks for updates on an annual basis or only when technicians visit the site. Paradoxically, the same isolation that protects equipment against some types of attacks results in longer exposure to emerging risks and increased maintenance expenses. If anomalies develop, diagnosing and rectifying problems may require site visits that take financial resources and personnel away from day-to-day operations.
The AI platform offers brownfield assets like remote, intermittently connected compressor stations improved cyber resiliency, reducing the frequency of false positives and update costs. In fact, the machine-learning detection engine predicts and prevents malicious activity without the need for an update. The platform can be installed on a Monday, receive no updates all week, and remain an effective defense on Friday—even if novel threats emerge in the middle of the week. As a result, the platform creates a frontline to detect and mitigate risks, creating a backstop for brownfield systems that are under-patched or unpatched.
Mitigating supply chain risks and insider threats. Refineries and processing plants are dynamic environments, with routine plant personnel and people from many different vendors moving in and out of the site daily. The risk of someone plugging a USB key into a computer and introducing—either accidentally or maliciously—some piece of malware can be quite high. This risk only increases during a plant turnaround or outage, when large numbers of unfamiliar people from OEM providers and maintenance crews will have physical access to network endpoints throughout the facility. Whether the attack originates from within or outside, one or more plant processes may be adversely affected—either by experiencing a drop in output or performance or failing altogether. And because the owner’s legacy antivirus and cyber protection systems are intentionally offline, so that software updates or changes can be applied, a failure on startup may be hard to diagnose. Was a failure the result of a failed mechanical repair or upgrade, misconfigured controls software, or malware creeping in unnoticed?
Once again, the platform helps avoid these scenarios with its machine-learning functionality. When first installed, the platform is placed in observer mode, as it learns the refinery or plant processes. Once the asset owner’s and OEM’s engineering teams are convinced that the platform understands the difference between routine operations and a cyberthreat, it is taken out of observer mode to track and control normal operations—without the risk of shutting down a critical component of the operation.
During a turnaround, shutdown, or outage, the platform can be placed back into observer mode, where it continues to track and report non-routine events or potential cyberthreats. But rather than shutting down the system and delaying the upgrade when it identifies an unfamiliar piece of software, the platform records and reports the event—including the precise computer or system where it arose—to the operations team, who can investigate further. As a result, the operations team can quickly go to that location, confirm the threat, and resolve the situation—before the threat spreads to other systems or becomes a more serious issue.
The MIT report highlighted several energy companies, which are implementing such fit-for-purpose digitization advances into their asset cybersecurity initiatives. Argentina’s Pampa Energía, for example, began executing a multiyear action plan in 2019, aimed at facilitating and promoting security by design, which will enable the secure digital transformation of its operations. Current digitalization efforts are directed toward optimizing performance and condition-based maintenance, including implementing an online monitoring program that links operations, maintenance, and plant experts, with the support of AI and machine-learning software. In 2020, the company rolled out a service for monitoring cybersecurity events to ensure that OT-related security incidents are correctly identified, analyzed, mitigated and reported. The data are then rolled into an automated dashboard, which stakeholders use to make faster and more informed security decisions.
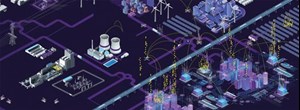
Creating collective defense in heterogeneous assets. Many asset owners face a great deal of heterogeneity in their install bases, with a mix of older and newer equipment, distributed and centralized systems and many different OEMs and third-party vendors on the premises, Fig. 3. Given this incredibly complex mesh of IT, OT, equipment, and personnel, most asset owners enact strict risk policies that cascade out to cover every asset in the field or plant and their edge computing architecture. For example, a common risk policy typically requires creating real-time connectivity all the way down and across the supply chain, with the ability to monitor that connectivity and track communication flows. Historically, the ability to deploy this policy out across the asset (or across multiple assets in the organization), while also being able to fine-tune or update risk postures in real time, has proven incredibly challenging.
But with the AI-enabled cybersecurity platform installed across the asset base, owners can seamlessly implement their policy-level measures and make updates in real time. The platform also allows for profiles to be linked from the higher-placed operational level down to the code level, and to be implemented and upgraded across distributed environments. The platform helps asset owners manage risk without hindering their productivity. For example, the platform allows for tuning security measures like multi-level password entry to ensure robust defense without slowing down work activities. Logging into a site multiple times to access information can be inefficient, particularly when a technician visiting the site for a limited number of hours needs to quickly access data from the edge architecture to resolve a problem.
CASE EXAMPLES
Spain’s Repsol has been building a collective defense and data management plan, based on AI, for the past several years. The plan incorporates digitizing operations to fundamentally change how projects are designed, how systems are built, the types of methodologies and technologies being used, the strategic vendors they partner with, and how they deliver IT services into their operations. Cybersecurity is a critical piece of this plan as well and the company has put in place a framework for cybersecurity that introduces AI-based technologies, new ways of working, and allocation of roles, controls, and policies such that cybersecurity is managed holistically across assets.
For many companies, the global Covid-19 pandemic accelerated efforts to digitalize more of their business operations, as the vast majority of their employees quickly shifted to working remotely. Companies moved to embed more big data, analytics, AI and machine learning functionality into their systems, to boost cybersecurity measures and to ensure reliable, collaborative workflows within teams separated by vast geographical distances or time zone differences.
Petrobras, for example, ramped up its digital transformation program to adopt more cloud computing, AI, and internet of things architecture in 2020. These initiatives not only bolstered the company’s IT security, but they also improved remote monitoring of critical OT systems and boosted efficiencies in a range of business processes.
Further measures to secure the energy infrastructure. As oil and gas companies shift to a risk management mindset, they need to implement cybersecurity strategies that go beyond just one service or product offering to instead incorporate services that help them mitigate cyberthreats at every link of their value chain. Consider the Colonial Pipeline attack earlier in 2021, in which the operator closed down operations and froze its IT systems on suspicion that their entire OT was breached. The ransomware attack forced the operator to proactively take most of its systems offline, operate one line solely on manual control, and scramble to mitigate the problem and restore full operational services as quickly as possible. This sudden and unexpected disruption resulted in real-world consequences, including supply problems for consumers, gas stations running dry, and panic buying of fuel in some areas of the U.S.
Siemens Energy developed its Managed Detection and Response (MDR) service, powered by Eos.ii, specifically to resolve cyberthreats like this—and minimize the real-world consequences of attacks that originate in an increasingly connected, virtual space. The MDR service combines cybersecurity expertise with detection technologies to collect raw IT and OT data from an owner’s operating environment and then translate and contextualize it in real time.
The service comprises three fundamental components: 1) protection, which is driven by AI and machine-learning systems like DeepArmor Industrial to automatically detect potential cyberthreats while notifying the owner’s operating crew and enacting mitigation measures to prevent more serious problems to process operations; 2) monitoring, which uses the Eos.ii platform to give potential cyberthreats a more visual context through the creation of a digital twin—a digital replica that models normal operations. Any deviations from normal operations are flagged and easily viewed by operations analysts, who can take action before a malicious command executes in the physical world; and 3) response, which uses the Precision Defense response method to deploy appropriate and targeted corrective measures to quickly recover from a cybersecurity incident. Implemented correctly, this method can fully contain and eradicate a threat, with the minimum possible disruption to production or plant operations.
Each of these components are AI powered. Taken together, they give the cybersecurity team greater visibility into what’s happening across each digitally connected node in the asset’s ecosystem. This visibility provides detailed context into the potential implications of a cyberthreat to individual assets and the system as a whole—allowing for more targeted, efficient, and cost-effective preventative measures to minimize malware-related downtime and optimize operations.
Cyber-defense strategy. As cyberthreats become more frequent, sophisticated and potentially damaging, operators across the energy chain will need to ensure that the protective measures for their increasingly connected infrastructure are equal to the task. Only through collaborative efforts—between operators, OEMs and startups—can we hope to stay ahead of cyber attackers and keep the energy value chain secure and operating at peak productivity and profitability.
- Aramco's upstream digital transformation helps illuminate the path toward excellence (June 2024)
- Adopting a holistic approach to cybersecurity (March 2024)
- Embracing automation: Oil and gas operators leverage new operational efficiencies (May 2024)
- The five A’s on the road to completions automation (May 2024)
- Improving drilling and logging operations with a new measurement platform (December 2021)