Digital smart products prove their value for processing technologies
To facilitate collaboration with end-users, NOV has created a set of digital products to accompany each of their processing technologies. These digital tools, which are included in a Process Intelligence Manager, have been deployed in the field since 2017. Pre-developed frameworks that are integrated into NOV’s open-source digital ecosystem MAX ensure a straightforward setup and give users the ability to operate seamlessly with any digital platform they may be using. This reduces the barriers for deployment and the time and resources required. These tools are flexible and can be configured according to specific needs to provide a wide range of functionalities that can be grouped into the following main categories:
New alert types. While traditional thresholding-based alerts only provide a notification when a parameter value exceeds a pre-defined limit, data analytics allow for the continuous search of outliers in the system’s behavior characteristics, identifying patterns that deviate from “normal.” This allows for the engineering of new alert types that provide additional insights into a technology’s behavior.
Digital process twins. By combining the different performance models from each subsystem and extending the data analytics to include correlations across subsystems, the digital process twin provides an accurate replica (or simulator) that is continuously updated with performance data to always remain relevant. This allows for the creation of more accurate performance models than generic counterparts or commercial simulator packages—which in turn allows for more accurate predictions of performance at future conditions.
Virtual sensors. Virtual sensors represent another application of predictive algorithms for measurements of parameters where physical sensors are not reliable or available. This approach combines fluid samples at discrete intervals, with the data from secondary sensors creating and continuously updating a “virtual sensor.” The model is recalibrated every time a fluid sample is taken, thus maintaining the accuracy of the model.
PREDICTIVE MAINTENANCE
A common starting point for applications of predictive data analytics has been to enable condition-based monitoring of pumps and other types of rotating equipment. Implementing predictive maintenance approaches helps to reduce downtime, optimize spare parts inventory, and maximize equipment lifetime. Diagnostics of components, such as bearings, are readily available, in addition to the health status of the equipment from prognostic models. As vibration levels increase, for example, the model outputs the probability of the cause from a list of known failure modes. When a failure mode is clearly identified, the remaining useful life can be evaluated and will allow maintenance tasks to be optimized.
NOV has applied this same approach for process technologies, specifically, using predictive maintenance to increase the availability of process systems. As an example, the company deployed data analytics to generate new alert types for the fouling detection for membranes in seawater treatment systems, Fig. 1. The system’s availability is critical, as it purifies water to be reinjected for improving the recovery of the oil reservoir. With more than 10 years of offshore operational experience and data trend observation, the most common fouling / upset events for such systems can be identified clearly. It also has allowed for the establishment of the characteristics of different types of build-up on the membranes (colloids, scale, and biological matter).
The early detection of these events is the key to enabling a proactive approach to operations. The built-in ability to differentiate between different types of fouling on membranes allows for recommendations of best-suited mitigation procedures. This reduces cleaning time and increases the lifetime of the membranes, hence improving availability and reducing the OPEX of the system.
PROCESS OPTIMIZATION THROUGH DATA ANALYTICS
Digital products for processing technologies should include process optimization functionality in addition to predictive maintenance. A typical control system already offers monitoring functionalities for process technologies, provides alarms, and takes corrective action when conditions deviate from normal. However, process optimization is not a normal feature.
As an example, consider a crude oil desalting process where electrostatic treaters are set up in series. Low-salinity water is mixed into the salty crude, followed by electrostatic separation of dispersed water droplets to remove residual salt water from the crude oil prior to refining. A sketch of the process units and the flow diagram is shown in Fig. 2.
Although electrostatic desalters do not have numerous operational variables to adjust, the recycle of water streams of different salinities makes the system quite difficult to optimize. Freshwater injection rate, water recycle rates, voltage set point of power units, mixing valve opening, demulsifier injection concentration, and heating input are common variables that are defined in the design stage or during start-up of the plant. Conventional operational optimization typically involves adjusting setpoint variables one by one and observing the resulting change in measured output variables.
Even the most experienced operator or technology expert on electrostatic treaters will struggle to provide the optimal operational set point, where the specifications for oil residual water content, salinity, and produced water quality are achieved with minimal impact to utilities and the environment. Water droplet dispersion behavior is difficult to predict because of the presence of naturally occurring surfactants in the crude oil and process parameters that influence each other. As a result, optimization is challenging by a conventional approach.
As an example, to reduce the crude oil salinity, the operator may want to increase the pressure drop over the first stage mixing valve to increase mixing efficiency of low-salinity water. However, this could increase the water carryover from the first-stage electrostatic desalter, as water droplets produced in the mixer are smaller. The efficiency of the washing process upstream the second stage is consequently affected, as there are smaller droplets and more water to be mixed with the same quantity of freshwater. This could impact the performance of the second-stage electrostatic desalter in terms of its water removal efficiency. As a consequence, the final oil quality and the salinity of the separated water being recycled to the first stage mixer (which was adjusted in the first place) would also be negatively impacted.
The Process Intelligence Manager for desalting of oil provides the operator with valuable insights on how to control and optimize this complex salt balance. The digital process twin utilizes data from the actual installation for “machine learning” to improve the simulation capabilities of the tool. In addition to typical field data, measurements of the residual water content and salinity in the crude is essential.
When trained with operational experience, the prediction tool has the potential to quantify the effect of varying operational conditions with better accuracy and relevance than what general theoretical models can offer alone. The tool can provide warnings to the operator when some adjustment to operational variables is recommended. Moreover, the prediction tool lets the user study how changes to various parameters influence the desalter’s operation, thus helping to find an optimum combination that can be confidently applied in the field.
OPERATIONAL SUPPORT WITH VIRTUAL PRESENCE
A significant benefit of deploying digital solutions is the ability to facilitate a “presence-in-the-field” of product specialists and subject matter experts, independent of their geographical location. Our experience is that technology specialists will discover additional areas for process improvement that an operational engineer might miss. Access to live process data and advanced data analytics reduces the need for offshore supervision and provides unique performance optimization opportunities for the facility.
The benefits of this approach were illustrated during a recent data review of a glycol regeneration system. Triethylene glycol (TEG) is commonly used to remove water from produced hydrocarbon gas. NOV reviewed six months of production data, which were loaded into a pre-developed framework on the MAX digital platform, together with corresponding laboratory results from fluids samples. Subject matter experts then compared these data sets with the system design parameters, including performance guarantees, and key performance indicators (KPIs). This allowed for a quick validation of process performance and informed new recommendations to further improve operational conditions.
A common KPI for the gas dehydration system is the overall TEG losses. Such losses can be calculated from time series of the surge drum level data during six months of operation, Fig. 3. During this time, a total of 14 sub-periods of normal operation were identified, separated by periods of system performance testing. The TEG losses were calculated for each of the periods of normal operations— and in all cases were confirmed to be below the specified system requirements (process guarantee).
A data review of a recently installed process system was expected to confirm that the TEG regeneration system was operating as originally designed. However, some points for improvement were identified, which helped confirm the benefits of providing an equipment manufacturer with detailed performance data. Furthermore, with the digital support infrastructure now in place, any future issues can quickly be analyzed with minimal resources, allowing for a rapid and cost-efficient response.
ENABLING NEW COMMERCIAL MODELS
One of the most common commercial setups within the oil and gas industry is for the end-user to contract an engineering and procurement company with the responsibility for the overall system engineering and fabrication, including contracting sub-suppliers for various subsystems and technologies. While this model ensures a clear definition of responsibilities during the engineering and fabrication phases, it may pose various challenges during the competitive tendering, as there could be a too-strong focus on providing a system that satisfies the project requirements at the lowest possible capital expenditure (CAPEX).
When such a system is put in operation, the choices made during the previous project phases to reduce CAPEX might, in some cases, result in increased OPEX, ultimately yielding an increase in total expenditures (TOTEX = CAPEX + OPEX). An example of such impact is the risk of increased chemical consumption if equipment is designed aggressively or increased manpower requirements are required if the level of automation and instrumentation is low.
To reduce the potential of overall cost increases, the concept of performance-based contracts has been proposed. Here the engineering companies and sub-suppliers (including equipment manufacturers) are awarded according to a risk and profit-sharing commercial structure, which rewards them for reducing and penalizes them for increasing both capital and operational costs. As a result, the focus of financial incentives is adjusted beyond the engineering and fabrication phases.
A common baseline is required to which the system performance can be evaluated. If a system or key technology is not satisfying the required process guarantee, it should be possible to quickly evaluate why—is it the result of improper design or because of how the system is operated? A reliable digital twin will create the necessary common basis that different parties can use to baseline system performance, which is ultimately fundamental to enabling performance-based contracts.
VIRTUAL SENSORS WHEN DATA ARE NOT AVAILABLE
A typical concern with introducing digital products for processing technologies is the lack of sufficient sensors in the facility. Without continuous data input, the value of a digital tool is limited. Many instruments in the process system are not always fully exploited for process optimization. Advanced online characterization of process fluids would be nice in some cases, but there could be alternatives when this is not feasible.
Virtual sensors can be generated to measure parameters when physical sensors are not installed or unavailable in the specific process plant, or when such online instruments are coming up short for various reasons. For example, virtual sensors can be adopted for the condition monitoring of monoethylene glycol (MEG), where a simulator is developed to describe the build-up of ions and precipitation of solids in the reclamation system.
MEG is used for hydrate inhibition of flowlines and is processed with the aqueous part of the production fluids, including formation water. Glycol is recovered in a process including reclamation, where salts and non-volatiles are separated from the glycol by flashing. Although the process aims to concentrate contaminants, high soluble salts, and the base form of organic acids, it is essential to control the accumulation in the flash separator bottom. Accumulation above a threshold value will increase the viscosity and boiling point of the fluid, which reduces the performance of the flash separator and the associated recycle loop.
Moreover, uncontrolled accumulation will increase the risk of equipment blockages and breakdown. For applications with large concentrations of these compounds, optimal performance of the reclamation process is ensured by frequent fluid sampling and laboratory analyses, which define how much excessive organic acid and high-solubility salts should be removed, Fig. 4. Frequent draining may increase MEG losses, whereas infrequent draining increases the risk of operational upset.
To reduce operational expenses and facilitate low-manned operations, the MEG Process Intelligence Manager is configured with a virtual sensor that predicts the accumulation of key ions and precipitation of solids in the reclaimer. The simulator is correlated to available secondary sensor data on the feed to the reclaimer and calibrated to results from actual fluids measurements taken at discrete intervals. This allows for continuous updates to the model between fluids samples, based on the input data from the secondary sensor. Every time a fluids sample is taken, the virtual sensor is calibrated, such that its accuracy is maintained. The time remaining before initiating reclaimer liquid dump and the volume to be drained can be estimated, based on the predefined thresholds for the salts and organic acids.
CONCLUSIONS
Data analytics will help improve condition-based monitoring and operation of processing technologies. Examples are provided, showing how tools with different features can be applied to systems for seawater treatment, saltwater removal from crude oil, water removal from gas, and monoethylene glycol reclamation.
Other processing technologies will benefit from similar analytical tools. Operational optimization of produced water treatment systems can be demonstrated. Increased monitoring and diagnostics can also improve reliability and performance of sand-handling operations, thus increasing availability of the facility, and maximizing produced water injectivity. Both are essential for operational success. We believe applications like those presented here will become a standard offering that accompanies processing equipment deliveries in the future.
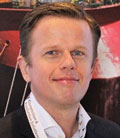
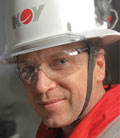
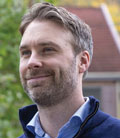
- ESP challenges in an EOR project after breakthrough: An analysis of continuous improvement for ESP designs towards the reduction of OPEX (June 2024)
- Aramco's upstream digital transformation helps illuminate the path toward excellence (June 2024)
- Explaining sand erosion in oil and gas production (June 2024)
- Rethinking sand management for optimized production (June 2024)
- Leveraging artificial lift to optimize production (June 2024)
- New technology allays permanent magnet motor safety concerns, enables better ESP performance (June 2024)