DAVID HABERLAH, FEI Company; and MIKE GARRICK, Halliburton
A joint development project undertaken by FEI Company (Hillsboro, Oregon), Halliburton (Houston, Texas), and Oil Search Limited (Sydney, Australia), has demonstrated a mobile, ruggedized workflow solution for advanced mudlogging services that provides near real-time data from drill cuttings to support time-critical, on-site decisions and the interpretation of downhole data. In the project, FEI’s Qemscan WellSite automated petrography solution (comprising sample preparation equipment, a scanning electron microscope (SEM) and energy dispersive X-ray spectrometers (EDS), designed to be deployed at the well site, was field tested at drilling sites in Papua New Guinea.
The remote site, in the inaccessible Southern Highlands within the complex and tectonically disturbed siliciclastic stratigraphy of the Papuan foldbelt, was chosen for the field test because it was both logistically and geologically challenging. The field test involved six months of on-site sample preparations, measurements and daily reporting for ›1,000 sample intervals collected from a gas injection well, a production well and an exploration well.
WORKFLOW
The integrated solution covers sample preparation, measurement, data processing, data analysis, reporting and archiving. A prime focus during the development and field test was to provide an automated, easy-to-operate workflow experience, from creating representative samples to providing consistent, reliable cuttings data of unprecedented detail. The workflow was extensively tested. The single longest continuous run by Halliburton staff lasted 34 days.
SAMPLE PREPARATION
In this system, sample preparation is different from conventional and other advanced mudlogging requirements. Drill cuttings are collected, wet-screened, dried, embedded in epoxy resin and cross-sectioned by a diamond saw, ultimately revealing 2-D sections of cuttings particles, as produced by the drill bit in their original sizes and shapes. As a result, there is no bias introduced by physical size fractionation or by reducing cuttings to powder, as required, for example, by x-ray diffraction (XRD), x-ray fluorescence (XRF) or laser-induced breakdown spectroscopy (LIBS).
Sample preparation, from cuttings collection to a measurement-ready sample block, took on average ~25 min. This provided enough time for operators to prepare samples and, in parallel, set up, run, process and report measurements. During the 12-hr single operator shifts, staff prepared an average 25 samples. However, they were able to prepare twice that number of sample blocks during drilling downtime, when processing a cutting samples backlog from previous drilling.
The onsite sample preparation workflow provides a convenient, efficient solution to sample handling and archiving. The measured blocks can be stored easily and take up less space than conventional bags of dry cuttings. If required, resin-mounted cuttings can be re-measured at a later time. The onsite sample preparation is a major leap from existing lab-based preparation procedures, which typically take up to two days, and is optimized for preparation of large batches of samples.
MEASUREMENT
Sample blocks can be loaded into the SEM’s vacuum chamber and measured, either one-by-one, or as batches of up to six blocks. Measurement parameters and workflow were optimized to minimize measurement times without compromising mineral and textural identification, and overall measurement statistics. Operating parameters in the field test targeted an average measurement time of ~30 min, to provide time-critical data within an hour from sample collection.
The mineral identification protocol for the workflow is based on the latest Qemscan Spectral Analysis Engine (SAE) element concentration approach. A custom Species Identification Protocol (SIP) was developed for drill cuttings in siliciclastic sequences capable of accurately discriminating more than 30 mineral groups, including clay minerals and micas, such as kaolinite, smectites, chlorite, illite and glauconite; solid solution series of feldspars; and trace minerals, such as pyrite, rutile and zircon. All mineral definitions are based on elemental ranges and ratios, and backscatter electron intensity (BSE) thresholds, and take into account the challenging sampling environment characterized by contamination from oil and drilling fluid additives.
The average measurement time in the field test was ~35 min, ranging from ~25–45 min., depending on the sample’s packing density. Measurements could keep up with the general drilling speed, resulting in an average 23 measurements per day. The average raw data generated per single sample interval is based on more than 300,000 spectra, collected on more than 2,000 genuine drill cuttings.
Setup and calibration of the workflow are fully automated. Measurements are started via a software interface developed to provide a single-click solution to system calibration—including e-beam alignment, BSE, EDS and SAE calibration. In the field test, all measurements and routine maintenance were successfully performed by staff without advanced SEM training. Maintenance included changing the tungsten filament.
DATA PROCESSING
Measurement of raw data is subsequently processed by offline software. Data processing can be separated into: 1) image-based operations performed on the stitched image of the measurement area, and 2) particle-based operations classifying individual particles into similar categories.
Despite wet-screening, most drilling intervals showed considerable contamination with drilling mud. Contamination by fines attached to cuttings was effectively removed by applying a set of digital screens to the raw data, including image-based field stitching, touching particle separation, size filter, and a boundary phase processor.
Some sample intervals were characterized by large contributions of Baracarb engineered ground marble, which is added to drilling mud as a borehole strengthener to prevent fluid loss, and by swarf generation from the drillstring. The workflow’s particle-by-particle classification was used to apply a set of contextual screens, separating additives from cuttings. As a result, data could be generated from genuine cuttings originating from the drilled rock, Fig. 1. Each data processing step, including digital and contextual screening, is documented in the metadata and fully reversible.
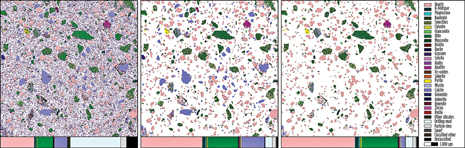 |
Fig. 1. Contaminated sample, demonstrating the impact of digital and contextual screening to improve the relevancy of reported data: wet-screened sample as measured (left); digitally screened sample (center); contextually screened sample (right). |
|
Particle classification was then applied to sort genuine drill cuttings into lithology classes defined by shared mineral composition and textural attributes. Cuttings classification is interactive and data-driven, developing a protocol that sorts the cuttings mix encountered within the sample interval into discrete, meaningful lithology categories. Cuttings classes are a snapshot of subsurface lithology, and take full account of regional basin geology, drilling practices and any other information required by the wellsite geologist, Fig. 2. Lithology classes carry potentially valuable information, which has not been applied previously to onsite interpretation of changes in subsurface geology and calibration of downhole data.
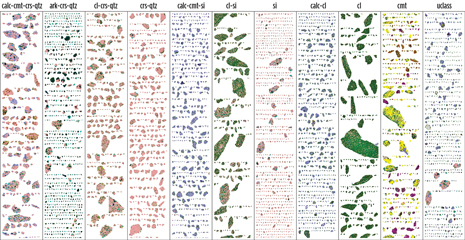 |
Fig. 2. Image of drill cuttings sorted into 10 lithology classes. |
|
The full set of detailed reports on mineral and lithology composition—including percentage cuttings return, average cuttings and grain size trends, the presence of tracer minerals, clay typing, and calculated matrix density-—was typically available within ~1 hr from sample collection at the shakers. Log data could be updated every 40 min.
RESULTS/DATA APPLICATIONS
During the field test, more than 1,000 samples were collected, prepared and measured. The largest continuous sampling sequence comprised 728 samples, collected in 34 days from one well. The following results are excerpts from this dataset, including ~200 million individual spectra from 1 million genuine cuttings.
System data are unique, in that images (individual compositional maps of cuttings) form the basis for all numerical and graphical reports. Qemscan reports can be generated for any selected sample interval, lithology class or even individual cuttings. For this description, drilling depth sections defined by drill bit size were selected as the basis for reporting results.
PARTICLES TO CUTTINGS
The first level of information provided by wellsite data includes reports on overall composition of particles within a sample sequence. In addition to drill cuttings, these can include cavings, swarf, additives and casing cement. Reports include percentage return of cuttings and their average size and shape (a useful measure of drill bit performance), in-situ stress fields and borehole condition. The left column of Fig. 3 presents relative contributions of various components in the raw data from the wet-screened sample. Note the significant contribution made by drilling mud particles.
CUTTINGS TO LITHOLOGY
The second level of information includes reports on the composition of representative drill cuttings within a sample sequence. In Fig. 3, removal of contaminants by digital (middle column) and contextual (right column) screening significantly impacts modal mineralogy. The reported mineral stratigraphy on genuine cuttings is shown to be more relevant and sensitive to changes in subsurface lithology than the whole sample composition.
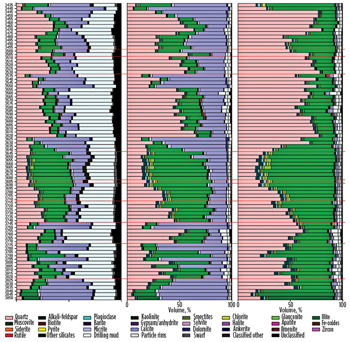 |
Fig. 3. Comparison of modal mineralogy results for wet-screened raw data (left); digitally screened data (center); and, final contextually screened data reporting on genuine cuttings (right). |
|
Reports can quantify the mineralogy of clays; discriminating swelling (e.g. smectites) from non-swelling (e.g. chlorite), and radioactive (e.g. illite) from non-radioactive (kaolinite) clay minerals. In addition, the presence of trace phases, such as pyrite, apatite, heavy minerals and tracer minerals, was monitored for downhole data calibration and well correlation. In addition to mineralogy, average mineral grain sizes were reported as equivalent sphere diameters. In the field test, quartz grain sizes were plotted as an independent means to highlight fining and coarsening trends in the siliciclastic sequence. The cuttings matrix density calculated from mineral reference data was reported and compared to downhole bulk density. As discussed in the data processing paragraph, drill cuttings were sorted into discrete lithology classes, based on similar mineral composition and textural attributes.
LITHOLOGIES TO PROPERTIES
The third level of information provided by Qemscan wellsite data includes detailed reports on cuttings composition and texture within individual lithology classes. Lithology-based reports include average modal mineralogy, quartz grain size distribution, average cuttings and quartz grain sizes, matrix density, and total number and volume contribution of classified cuttings for selected sample intervals. Figure 4 presents a report for claystone, one of ten basin-specific lithology class reports.
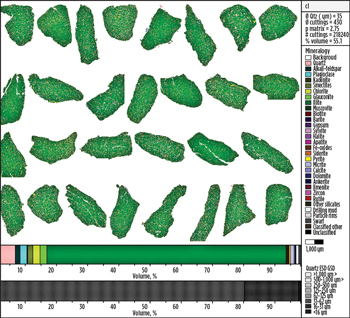 |
Fig. 4. Property sheet for lithology class ‘cl’ (clay), based on combined well cuttings. This cuttings class comprises illite-rich claystone cuttings. |
|
A set of 11 report templates was developed to provide consistent field test data reporting. These reports can easily be adapted and expanded to meet individual requirements:
-
MapView–petrographic image for each sample
-
MinLog–modal mineralogy (volume or mass %) for selected sample sequence, including clay mineral, feldspars, heavy and trace mineral quantification
-
LithoView–cuttings images for defined lithology classes
-
LithoLog–lithology classes contributions (volume or mass %) for selected sample sequence
-
DensityLog–calculated matrix density for selected sample sequence
-
SizeLog–average quartz grain size (or cuttings size) for selected sample sequence
-
LithoMin–modal mineralogy (volume or mass %) for selected lithology classes
-
LithoProp–lithology class properties for selected sample sequence
-
LithoQtzGSD–average quartz grain-size distribution for selected lithology classes
-
Return–percentage return (volume or mass %) of drill cuttings and contaminants/additives for selected sample sequence
-
Stats–measurement statistics for selected sample sequence.
PROPERTIES TO INTEGRATED LOGS
Selected wellsite data were exported as numerical log data and imported into Oilfield Data Manager (ODM) third-party integration and interpretation software, designed to store, manage and interpret geological well data. Within the ODM program, data were juxtaposed with data from selected downhole measurements, including rate of penetration; gamma radiation; calliper; bulk density; resistivity (not displayed); and neutron porosity logs, Fig. 5. The example from the integrated log highlights aspects of the added information obtained by this system’s data.
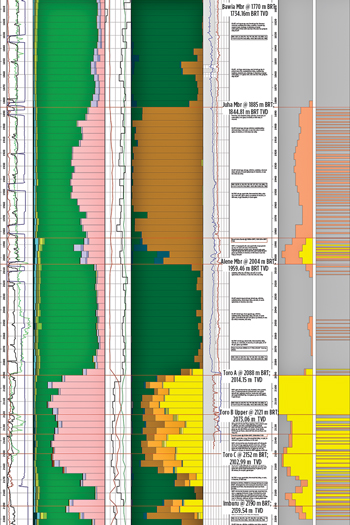 |
Fig. 5. Integrated ODM log juxtaposing QEMSCAN data with selected downhole data and the original Formation Evaluation Log, including written remarks by the mud loggers, the conventional cuttings log, and the final interpreted lithology log by the wellsite geologist. |
|
Quantification of hydrous micas, such as glauconite, supports interpretation of the neutron porosity log, by calibrating the inferred clay reading, and interpretation of the resistivity logs, by calibrating inferred formation water content. Volume contributions of alkali feldspars, as well as non-radioactive clays (kaolinite), support interpretation of the gamma-ray log response. Interpretation of resistivity logs is supported by showing the presence or absence of pyrite and clay grain coatings. Quantitative Qemscan clay typing further assists in interpreting changes in drill bit ROP and in the borehole diameter, by discriminating swelling (smectites) from non-swelling clays (e.g. illite).
In Fig. 5, the Alene member is characterized by a sudden increase in smectites and intervals with high glauconite content. Identification of clays and clay types also assisted in the deterministic petrophysical interpretation of the drilled intervals, particularly with respect to the volume of clay parameter. It is anticipated that the mineral identification information, extracted from the data, will assist in the derivation of a more accurate multi-mineral model for usage in an optimal solution, petrophysical interpretation.
The lithology log corresponds well to geological formation tops. Intervals characterized by claystone, siltstone and sandstone are clearly distinguished. In the given example, siltstone of the Juha member is identified and discriminated from the Toro formation's sandstone reservoir intervals, despite overall similar mineralogy. The lithology log also provides detailed information on lithology associations with carbonate cementation. Here, calcite cementation in claystone of the Bawia member is contrasted with calcite cementation in siltstone of the Basal Juha sands. Individual Toro members are clearly identified as sandstone intervals, separated by an increase in clay-rich siltstone. Qemscan data integration with downhole data shows considerable potential, but is still at an early stage. Using ODM software, different logs can be designed to highlight specific correlations, such as clay mineralogy with gamma-ray logs.
Wellsite log data were also compared with the original formation evaluation log provided by onsite surface loggers. Overall, the Qemscan lithology log compares well with conventional classification of cuttings into sand, silt and claystone, based on optical microscopy observations. Discrepancies in the amount of sand, silt and claystone reported in the two logs can be partly explained by written remarks in the formation evaluation log, where inferences are made about contamination. The dominant lithology reported in the formation evaluation log generally has a stronger presence than reported in the Qemscan lithology log. This suggests differences between qualitative lithology descriptions and consistent quantitative lithology classification. The comparison also highlights the limited ability of conventional mud logging to describe clay mineralogy, presence of genuine carbonate cementation and the type of feldspars, all of which are combined in remarks.
CONCLUSIONS
Successful operation of the Qemscan wellsite petrography solution in a challenging onshore rig environment demonstrated the ruggedness of the mobile system and the sample preparation equipment. All operational challenges were resolved on-site without requiring shipment of additional consumables, tools or spare parts.
The Qemscan wellsite process was successfully integrated into 12-hr, workshift surface logging operations. Sample collection, preparation and measurement could keep up with drilling speed.
The field test demonstrated an ability to report near real-time data that can support time-critical, on-site decisions and downhole data interpretation. The field test also demonstrated that digital removal of contaminants, in addition to the wet-screening routinely performed by surface loggers, can provide relevant data on subsurface geology.
Associating Qemscan lithology log data with downhole instrument data and conventional cuttings descriptions shows good alignment between geological formation tops. Detail provided on clay mineralogy, feldspars and lithology associations with carbonates is unprecedented, and was used to improve interpretation of downhole data, in particular the gamma-ray and resistivity log responses. 
THE AUTHORS
|
DAVID HABERLAH is a senior applications scientist for automated petrography solutions at FEI Natural Resources in Brisbane, Australia. He graduated with an MSc degree in Physical Geography from the Free University of Berlin in 2004, and obtained his PhD in geology during 2009 at the University of Adelaide. From 2009 to 2010, he held a research fellow position within CO2CRC at the Australian School of Petroleum. Mr. Haberlah joined FEI in late 2010, and he supported application development in the Papua New Guinea field test.
|
|
MIKE GARRICK is a data engineer with Halliburton in the North Sea. He earned a BSc degree in geology from the University of Portsmouth in 2005, where he specialized in structural geology. He started working for Halliburton on various rigs as a surface logger in late 2006, and he was promoted to unit manager. Showing a keen interest in the development of advanced mudlogging solutions, Mr. Garrick assisted in the field test of the Qemscan wellsite solution in Papua New Guinea. |
|